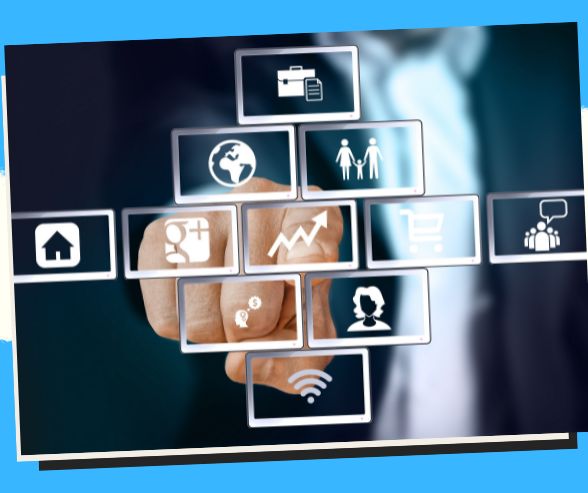
Bridging the Gap: How Semi-Supervised Learning Harnesses the Power of Both Worlds
Bridging data realms – delve into the realm where labeled and unlabeled data converge, guided by semi-supervised learning’s transformative approach to extracting insights from both worlds.
Bridging the Gap: Unveiling Semi-Supervised Learning in AI and ML 

In the realm of technology, Artificial Intelligence (AI) and Machine Learning (ML) continue to push boundaries and transform the way we perceive data and patterns. For aspiring and newbie IT professionals, delving into Semi-Supervised Learning isn’t just an exploration; it’s a journey into the world of bridging the gap between labeled and unlabeled data. In this article, we’ll embark on a captivating journey, unraveling the intricacies of Semi-Supervised Learning, showcasing how it combines the best of both worlds and illuminating its significance in reshaping the landscape of AI and ML.
The Essence of Semi-Supervised Learning: Merging Labeled and Unlabeled Data 

Semi-Supervised Learning exists at the intersection of supervised and unsupervised learning. While supervised learning relies on labeled data for training, and unsupervised learning explores patterns in unlabeled data, semi-supervised learning ingeniously combines both. It leverages the power of a limited set of labeled data to enhance the insights drawn from a larger pool of unlabeled data.
A Powerful Symbiosis: The Semi-Supervised Approach 

Semi-Supervised Learning capitalizes on the synergistic potential of labeled and unlabeled data:
- Labeled Data: A small subset of data points with known labels is used to guide the learning process.
- Unlabeled Data: A larger pool of data without labels provides additional context and information.
By tapping into the strengths of both labeled and unlabeled data, Semi-Supervised Learning transcends the limitations of scarce labeled data, offering more robust and generalized solutions.
The Mechanics of Semi-Supervised Learning 

Semi-Supervised Learning involves a delicate balance between supervision and autonomy:
- Initial Labeled Data: A small amount of labeled data is provided to the model, allowing it to establish some baseline understanding.
- Unlabeled Data Integration: The model processes the larger pool of unlabeled data, extracting patterns, relationships, and features.
- Semi-Supervised Training: The model uses both labeled and unlabeled data to iteratively update its internal representations, fine-tuning its understanding.
- Enhanced Predictions: The model’s performance is improved by utilizing the insights gained from the unlabeled data.
The beauty of Semi-Supervised Learning lies in its ability to harness the latent knowledge hidden within the vast ocean of unlabeled data.
Real-World Applications of Semi-Supervised Learning 

Semi-Supervised Learning finds practical application across various domains, revolutionizing how industries approach complex challenges:
1. Speech and Audio Analysis:
Semi-Supervised Learning aids in recognizing speech patterns and understanding context in audio data, even with a limited set of labeled speech samples.
2. Text Classification and Sentiment Analysis:
Analyzing a massive amount of text data becomes more accurate with the integration of unlabeled data. This enhances the precision of sentiment analysis and topic classification.
3. Medical Imaging:
In medical fields, Semi-Supervised Learning can detect anomalies in medical images. It combines labeled images of known conditions with unlabeled images to create a more comprehensive understanding.
4. Fraud Detection:
For credit card fraud detection, Semi-Supervised Learning can identify rare and previously unseen fraud patterns by learning from a combination of labeled fraud cases and unlabeled transactions.
5. Anomaly Detection:
In industries like cybersecurity, Semi-Supervised Learning can identify anomalies in network traffic by learning from both normal and abnormal data patterns.
Striding Toward Semi-Supervised Mastery: Tips for Beginners 

For aspiring and newbie IT professionals intrigued by Semi-Supervised Learning, here’s a roadmap to mastery:
1. Grasp the Basics:
Understand the foundational concepts of supervised and unsupervised learning to appreciate the significance of Semi-Supervised Learning.
2. Learn Algorithms:
Familiarize yourself with algorithms like Self-Training, Multi-View Learning, and Co-Training, which are tailored for Semi-Supervised Learning scenarios.
3. Dataset Exploration:
Practice Semi-Supervised Learning using publicly available datasets that include both labeled and unlabeled data.
4. Online Courses and Tutorials:
Engage with online courses and tutorials that focus on Semi-Supervised Learning techniques, providing both theoretical understanding and practical implementation.
5. Collaborate and Experiment:
Join online communities, participate in discussions, and collaborate with peers to learn from shared experiences and experiment with real-world datasets.
Embracing the Future: Your Role in the Semi-Supervised Story 

Aspiring and newbie IT professionals who delve into the realm of Semi-Supervised Learning are stepping into a domain of innovation and exploration. The fusion of labeled and unlabeled data transforms the landscape of AI and ML, empowering you to extract insights from data that were previously untapped. In a world where data is abundant but labeled annotations are scarce, your understanding of Semi-Supervised Learning is a passport to unlocking hidden knowledge. By embracing the Semi-Supervised approach, you’re becoming part of a transformative narrative that propels industries, research, and solutions forward, all while standing at the intersection of human-guided learning and data-driven autonomy.
Related Queries
Data Alchemy: Semi-Supervised Learning with Labeled and Unlabeled Data
Bridging Data Realms: Semi-Supervised Learning’s Approach
Synergy Unleashed: Semi-Supervised Learning’s Power
Dual Data Dynamics: Semi-Supervised Learning Unveiled
Fusion Empowerment: Magic of Semi-Supervised Learning
Learning Redefined: Semi-Supervised Approach
Hybrid Solution: Merging Data Realities in Semi-Supervised Learning
Illuminating the Unknown: Semi-Supervised Learning’s Path
Revolution of Insight: Semi-Supervised Empowerment
Unleashing Potential: Semi-Supervised Learning’s Art
Save/Share this story with QR CODE
Disclaimer
This article is for informational purposes only and does not constitute endorsement of any specific technologies or methodologies and financial advice or endorsement of any specific products or services.
Need to get in touch?
Feel free to Contact NextGenDay.com for comments, suggestions, reviews, or anything else.
We appreciate your reading. Simple Ways To Say Thanks & Support Us:
1.) GIVE A TIP. Send a small donation thru Paypal
Your DONATION will be used to fund and maintain NEXTGENDAY.com
Subscribers in the Philippines can make donations to mobile number 0917 906 3081, thru GCash.
3.) BUY or SIGN UP to our AFFILIATE PARTNERS.
4.) Give this news article a THUMBS UP, and Leave a Comment (at Least Five Words).
AFFILIATE PARTNERS
World Class Nutritional Supplements - Buy Highest Quality Products, Purest Most Healthy Ingredients, Direct to your Door! Up to 90% OFF.
Join LiveGood Today - A company created to satisfy the world's most demanding leaders and entrepreneurs, with the best compensation plan today.