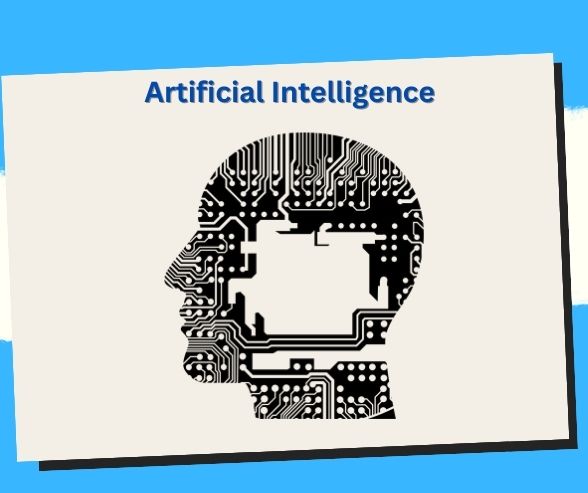
π©βπ» Ensuring Fair AI: The Crucial Role of Diverse Training Data ππ€
Learn how to ensure fair AI by understanding the significance of diverse training data in this comprehensive guide. ππ€ #FairAI #InclusiveData
The Quest for Fair Representation in AI Training Data π€π
In the age of artificial intelligence, our world is rapidly evolving. AI has become an integral part of our daily lives, from intelligent virtual assistants to self-driving cars. However, as AI systems continue to shape our world, a pressing concern arises the need for fair representation in AI training data. In this article, we will delve deep into the significance of fair representation, its challenges, and the solutions that aim to build a more equitable AI ecosystem. ππ
Unpacking Fair Representation π§
What is Fair Representation in AI Training Data?
Fair representation in AI training data means that the data used to train machine learning models should reflect the diversity and characteristics of the real world as accurately as possible. The goal is to ensure that AI systems do not inadvertently perpetuate bias or discrimination by learning from skewed or unrepresentative data.
The Importance of Fair Representation π
Fair representation is pivotal in the world of AI for several reasons:
1. Reducing Bias and Discrimination
Unfair representation in AI training data can lead to biased and discriminatory outcomes. When AI models are trained on data not representative of the diverse population, they may unintentionally discriminate against certain groups, exacerbating social disparities.
2. Enhancing Accuracy
A machine learning model’s accuracy and generalization depend on the quality of the training data. Fairly represented data provides a broader and more accurate picture of the world, allowing AI systems to make better predictions and decisions.
3. Avoiding Harm
Unfair representation can lead to harmful consequences. For example, if AI models are trained on data primarily from one demographic group in healthcare, they may provide inaccurate or potentially harmful medical advice to other groups.
The Challenges of Fair Representation in AI π§
Despite the importance of fair representation, achieving it is far from straightforward. Several challenges must be overcome:
1. Data Bias
Data bias is a fundamental issue in AI training data. It often arises from historical disparities and inequalities, as well as the biases of data collectors. AI models will inherit these biases if certain groups are underrepresented or misrepresented in the data.
2. Privacy Concerns
Balancing the need for diverse training data with privacy concerns can be challenging. Some data sources may contain sensitive information, and using this data while protecting individual privacy is a delicate task.
3. Data Availability
Sometimes, data for specific demographic groups may be scarce or hard to access. This scarcity can make it challenging to create diverse training datasets.
4. Labeling Bias
Human-generated labels for AI training data can introduce bias. Labelers’ biases or their lack of cultural sensitivity can lead to inaccurate representations.
Strategies for Achieving Fair Representation in AI Training Data π οΈ
While fair representation in AI training data is a complex task, several strategies and techniques can help in the pursuit of equity:
1. Diverse Data Collection
Diversity starts at the data collection stage. Collect data from various sources, regions, and demographics to ensure fair representation. This may involve partnerships with diverse communities or organizations.
2. Data Augmentation
Data augmentation techniques can help increase diversity in training data. These methods involve artificially expanding the dataset by generating new data points or transforming existing ones to create a more balanced representation.
3. Privacy-Preserving Techniques
Privacy-preserving techniques, like federated learning or differential privacy, allow organizations to collect data without compromising individual privacy. These approaches enable the use of sensitive data while safeguarding personal information.
4. Bias Mitigation Algorithms
Machine learning algorithms designed to mitigate bias can help counteract the effects of unfair representation in training data. These algorithms work to provide more equitable predictions and decisions.
Real-World Applications and Impact π
Fair representation in AI training data has far-reaching implications in various fields. Let’s explore some real-world applications and their potential impact:
1. Healthcare
In healthcare, AI can assist in diagnosis and treatment recommendations. Fairly represented training data ensures that AI models are equally proficient at diagnosing diseases and offering treatment options for diverse patient populations.
2. Finance
In the financial sector, AI-driven models help with credit scoring and investment recommendations. Fair representation in data ensures that these models do not favor one demographic group over another, promoting economic equity.
3. Criminal Justice
AI systems play a role in the criminal justice system, aiding in risk assessment, bail decisions, and sentencing. Fair representation helps ensure that the algorithms do not exacerbate existing inequalities in the justice system.
4. Education
AI is making its mark in education through personalized learning platforms. Fair representation in training data guarantees that these systems tailor recommendations to students from all backgrounds.
The Role of Regulations and Ethical Guidelines π
To promote fair representation in AI training data, regulatory bodies, and industry standards are emerging as crucial players in the quest for equity:
GDPR and Data Protection
The General Data Protection Regulation (GDPR) in Europe strongly emphasizes data protection and individual rights. GDPR encourages organizations to ensure fair representation of AI training data while respecting privacy.
AI Ethics Guidelines
Organizations such as the IEEE and the Partnership on AI have developed AI ethics guidelines that underline the importance of fair representation. These guidelines stress the need for transparency and equity in AI systems.
Federal Legislation
Several countries, including the United States, are exploring or enacting AI and data fairness legislation. These regulations aim to establish standards for the responsible use of AI, ensuring equitable representation.
The Path Forward: Towards a More Equitable AI Landscape π
The journey toward fair representation in AI training data is ongoing, but it is a path well worth traveling. As technology continues to transform our world, we are responsible for ensuring that AI systems reflect the diversity and inclusivity of our global society.
Technological Advancements
Advances in technology will undoubtedly play a role in addressing the challenges of fair representation. Machine learning models are becoming more adept at recognizing and mitigating bias in training data.
Public Awareness
Public awareness of the importance of fair representation in AI is growing. This awareness serves as a catalyst for change by holding organizations and developers accountable for their ethical responsibilities.
Ethical AI
The field of ethical AI is rapidly evolving, with researchers and ethicists actively working on innovative techniques and frameworks to achieve fair representation. Ethical considerations are becoming an integral part of AI development.
Collaborative Efforts
Stakeholders from all sectorsβgovernments, businesses, researchers, and advocacy groupsβrecognize collaboration’s importance in addressing AI bias. Joint efforts are essential to creating a more equitable AI landscape.
In Conclusion: Building a More Inclusive Future π
Fair representation in AI training data is not a mere aspiration; it’s a fundamental necessity. As AI continues to shape our world, we must take collective action to ensure equitable representation. Let us strive for an AI landscape that empowers and uplifts all individuals, regardless of their background or characteristics, fostering a more just and inclusive world. π€ππ
Related Queries
Creating Fair AI through Data Diversity
Inclusivity in AI Training Data
Diverse Data’s Role in AI Equity
Building Fair AI Models with Inclusive Data
Strategies for Fair Representation in AI
Balancing Bias in AI Training Data
The Power of Inclusive Datasets in AI
Inclusive Data Techniques for AI Fairness
Equitable AI through Data Inclusivity
The Future of Fairness in AI with Inclusive Data
Save/Share this story with QR CODE
Disclaimer
This article is for informational purposes only and does not constitute endorsement of any specific technologies or methodologies and financial advice or endorsement of any specific products or services.
π© Need to get in touch?
π© Feel free to Contact NextGenDay.com for comments, suggestions, reviews, or anything else.
We appreciate your reading. πSimple Ways To Say Thanks & Support Us:
1.) β€οΈGIVE A TIP. Send a small donation thru Paypalπβ€οΈ
Your DONATION will be used to fund and maintain NEXTGENDAY.com
Subscribers in the Philippines can make donations to mobile number 0917 906 3081, thru GCash.
3.) π BUY or SIGN UP to our AFFILIATE PARTNERS.
4.) π Give this news article a THUMBS UP, and Leave a Comment (at Least Five Words).
AFFILIATE PARTNERS
World Class Nutritional Supplements - Buy Highest Quality Products, Purest Most Healthy Ingredients, Direct to your Door! Up to 90% OFF.
Join LiveGood Today - A company created to satisfy the world's most demanding leaders and entrepreneurs, with the best compensation plan today.