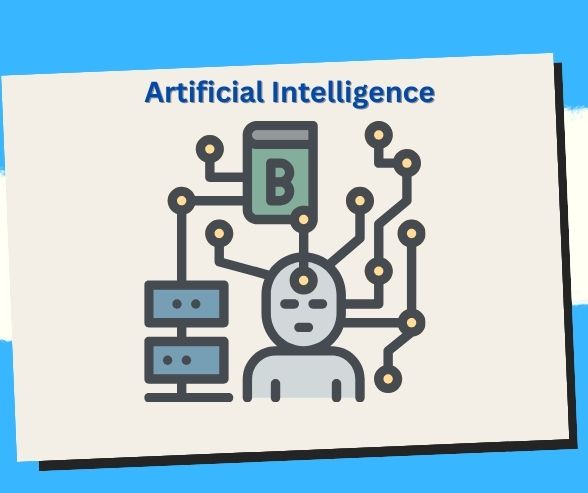
🚀 Breaking Barriers: Addressing Racial and Gender Biases in AI 👩🏽💻🧑🏿💻
Explore the pivotal journey of addressing racial and gender biases in AI. Learn how technology is reshaping to foster inclusivity and diversity. Dive into the conversation now. 🔗 #AI #Bias #Diversity
🌍 Addressing Racial and Gender Biases in AI: A Path to Equality and Fairness 🤖
In the Artificial Intelligence (AI) age, our reliance on algorithms and machine learning models has never been more pronounced. From personalized recommendations on streaming platforms to automating decision-making processes in industries like finance, healthcare, and criminal justice, AI is transforming the world. However, this transformation is not without its challenges, chief among them being the persistence of racial and gender biases in AI systems. In this article, we’ll explore the roots of these biases, their consequences, and the proactive measures being taken to eliminate them from AI technology.
🎯 The Pervasive Problem of Bias in AI
Bias in AI is not an inherent quality of the technology itself but rather a reflection of the data and processes used to train the AI models. It is this training data, often collected and curated by humans, that introduces bias into AI systems. The data can inadvertently carry societal prejudices, leading to AI models that make discriminatory decisions, often affecting marginalized groups more significantly.
Racial and gender biases are two of the most troubling forms of bias in AI systems. These biases can manifest in numerous ways, including:
1. Recruitment and Employment: Some AI systems used in hiring processes may inadvertently favor certain racial or gender groups, leading to discriminatory hiring practices.
2. Criminal Justice: Biased AI algorithms can affect sentencing recommendations and parole decisions, disproportionately affecting minority populations.
3. Healthcare: AI systems used in medical diagnosis may exhibit biases in their recommendations and treatment plans, potentially affecting the health outcomes of patients.
4. Finance: In credit scoring and lending, biases can result in unequal access to financial services based on race or gender.
🧐 Understanding the Origins of Bias
To effectively address racial and gender biases in AI, it’s crucial to understand their origins. These biases typically arise from three primary sources:
1. Training Data Bias: AI models learn from data, and if the training data contains historical biases, the model is likely to perpetuate those biases. For example, if past hiring data shows a preference for male candidates, the AI model may continue this trend.
2. Data Collection Bias: The data used for training humans is often collected by AI systems and can reflect their biases. This is especially concerning in facial recognition technology, where biased data can lead to misidentifications and misclassifications.
3. Algorithmic Bias: The algorithms can introduce bias due to their design. For example, an algorithm might prioritize speed or cost savings over fairness, leading to discriminatory outcomes.
🌐 The Impact of Racial and Gender Biases in AI
The consequences of racial and gender biases in AI are far-reaching and can exacerbate societal disparities. Here’s a closer look at some of the impacts:
1. Reinforcement of Stereotypes: Biased AI models can reinforce harmful stereotypes about certain racial and gender groups, perpetuating discrimination and inequality.
2. Discrimination: Discriminatory outcomes can lead to unjust treatment in various sectors, including hiring, criminal justice, and access to financial services.
3. Eroding Trust: Public trust in AI and the institutions using it can erode when biased outcomes come to light. This can hinder the adoption of AI in areas where it could provide significant benefits.
4. Legal and Ethical Issues: Biased AI systems can lead to legal and ethical challenges, as they may violate laws that protect against discrimination and bias.
📈 Steps Towards Eliminating Bias in AI
Addressing racial and gender biases in AI is a complex and ongoing endeavor. It involves multiple stakeholders, including AI developers, organizations, regulators, and the broader public. Here are some critical steps towards eliminating bias in AI:
1. Diverse and Representative Data: The foundation of unbiased AI systems is diverse and representative data. Efforts should be made to ensure that training data is inclusive and reflects the real world’s diversity.
2. Bias Mitigation Techniques: Developers can use various techniques to reduce bias in AI models, such as re-weighting data, adversarial training, and fairness constraints.
3. Transparency and Explainability: AI models should be designed to be transparent and explainable, allowing users to understand how decisions are made. This can help detect and rectify biases.
4. Regulatory Frameworks: Governments and regulatory bodies can play a crucial role in setting standards and regulations for AI, ensuring that it operates ethically and fairly.
5. Ethical Guidelines: Organizations should establish ethical guidelines for using AI, emphasizing fairness and equality as core principles.
6. Public Awareness: Increasing public awareness about bias in AI and its consequences can lead to greater scrutiny and accountability.
🌟 Success Stories and Ongoing Challenges
While addressing racial and gender biases in AI remains a significant challenge, there are also success stories that show it is possible to make progress. For example:
1. Facial Recognition: Tech companies have made strides in improving the accuracy of facial recognition technology for various skin tones and gender identities.
2. Criminal Justice: Some jurisdictions have reevaluated their use of AI in the criminal justice system, leading to more equitable sentencing and parole decisions.
3. Bias Detection Tools: The development of bias detection tools has allowed organizations to identify and rectify biases in their AI systems more effectively.
However, challenges persist. AI is a constantly evolving field, and new forms of bias emerge as new technologies and applications emerge. It’s a dynamic battle that requires vigilance and adaptability.
🌏 The Global Perspective
The issue of racial and gender biases in AI is not confined to a single region or country. It’s a global challenge, which requires international collaboration and shared best practices.
Organizations like the United Nations and the World Economic Forum actively work on AI ethics and promote responsible AI use. They emphasize the importance of AI which respects human rights and promotes equality.
🚀 Looking to the Future
As AI continues to advance and integrate further into our lives, addressing racial and gender biases remains a top priority. Achieving unbiased AI systems is not just a matter of ethics; it’s about creating a fair and just society that benefits everyone, regardless of race or gender.
The journey towards unbiased AI is ongoing and demands the collective effort of governments, organizations, researchers, developers, and the public. It’s a commitment to a future where AI is a force for equality and fairness and empowers rather than discriminates.
🤝 The Role We All Play
In conclusion, addressing racial and gender biases in AI is a shared responsibility. Whether you are an AI developer, a business leader, a policymaker, or a concerned individual, you can contribute to eliminating bias in AI.
By actively working towards unbiased AI, supporting regulations that ensure fairness, and raising awareness about the importance of ethical AI, we can collectively create a future where AI technology reflects the diversity and equality we value in society. Together, we can pave the way for a world where AI truly serves all, irrespective of race or gender. 🌎🌟🤝
Related Queries
Addressing racial and gender biases in AI
Quest for equality and fairness in AI
Tackling AI bias head-on
Creating ethical AI for everyone
Breaking barriers in AI
Diversity in technology
Confronting AI bias
Guarding against AI biases
Inclusive tech landscape
Cracking the code on AI bias
Save/Share this story with QR CODE
Disclaimer
This article is for informational purposes only and does not constitute endorsement of any specific technologies or methodologies and financial advice or endorsement of any specific products or services.
📩 Need to get in touch?
📩 Feel free to Contact NextGenDay.com for comments, suggestions, reviews, or anything else.
We appreciate your reading. 😊Simple Ways To Say Thanks & Support Us:
1.) ❤️GIVE A TIP. Send a small donation thru Paypal😊❤️
Your DONATION will be used to fund and maintain NEXTGENDAY.com
Subscribers in the Philippines can make donations to mobile number 0917 906 3081, thru GCash.
3.) 🛒 BUY or SIGN UP to our AFFILIATE PARTNERS.
4.) 👍 Give this news article a THUMBS UP, and Leave a Comment (at Least Five Words).
AFFILIATE PARTNERS
World Class Nutritional Supplements - Buy Highest Quality Products, Purest Most Healthy Ingredients, Direct to your Door! Up to 90% OFF.
Join LiveGood Today - A company created to satisfy the world's most demanding leaders and entrepreneurs, with the best compensation plan today.