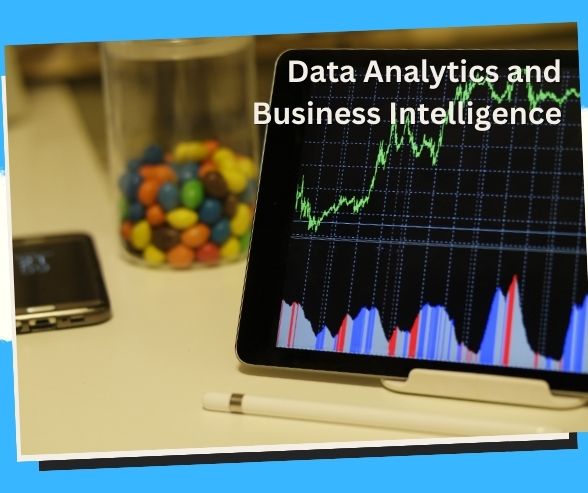
Boost Sales and Customer Loyalty: The Power of Predictive Analytics 
Boost sales and foster customer loyalty with the power of predictive analytics. Uncover how this technology is transforming retail businesses and creating satisfied customers.
Transforming Retail with Predictive Analytics 

The retail industry has always been dynamic and fast-paced, with changing consumer preferences, market trends, and technological advancements. In recent years, the game-changer thatβs been helping retailers stay ahead in this competitive landscape is predictive analytics. This cutting-edge technology isnβt just a buzzword; itβs the key to understanding customer behavior, optimizing inventory, enhancing the shopping experience, and ultimately, increasing profitability.
In this article, weβll take a deep dive into the world of predictive analytics in retail. Weβll explore what it is, how it works, and, most importantly, how itβs transforming the retail sector. So, grab your shopping cart, and letβs embark on a journey through the aisles of predictive analytics in the retail world!
Understanding Predictive Analytics
Before we dive into how predictive analytics is revolutionizing the retail industry, letβs establish a foundational understanding of what predictive analytics is and how it works.
Predictive analytics is a subset of data analytics that uses historical data, statistical algorithms, and machine learning techniques to identify the likelihood of future outcomes based on past data. It goes beyond descriptive analytics, which simply reports what has happened, and diagnostic analytics, which explains why it happened. Predictive analytics, on the other hand, is all about forecasting what will happen next.
How Predictive Analytics Works:
- Data Collection: The first step in predictive analytics is gathering relevant data. This data can come from various sources, including customer transactions, website interactions, social media, etc. The more data available, the better the predictions can be.
- Data Cleaning and Preparation: Once the data is collected, it must be cleaned and prepared for analysis. This includes handling missing values, removing outliers, and transforming the data into a usable format.
- Model Development: Predictive models are built using statistical and machine learning techniques. These models can be as simple as linear regression or as complex as neural networks. The choice of the model depends on the specific prediction task.
- Training the Model: The model is trained using historical data, where it learns the patterns and relationships in the data. This step is crucial because the modelβs performance depends on the quality of the training data.
- Making Predictions: Once the model is trained, it can start making predictions on new, unseen data. For example, in retail, it can predict which products a customer is likely to purchase or when a particular item is likely to go out of stock.
- Evaluation and Iteration: Predictive models are imperfect, and their accuracy must be evaluated. If the predictions are not satisfactory, the model can be refined, and the process is repeated until the desired level of accuracy is achieved.
Use Cases in Retail:
Predictive analytics can be applied to various aspects of retail, including:
- Demand Forecasting: Predicting customer demand for specific products, allowing retailers to optimize inventory and prevent stockouts or overstock situations.
- Customer Segmentation: Identifying different customer segments based on behavior and demographics, enabling personalized marketing and product recommendations.
- Price Optimization: Determining the optimal product prices to maximize profits and stay competitive.
- Inventory Management: Predicting when and how much stock needs to be replenished, reducing carrying costs, and minimizing losses due to excess inventory.
- Churn Prediction: Anticipating when customers are likely to stop shopping with a retailer, enabling proactive retention efforts.
- Fraud Detection: Identifying potentially fraudulent transactions in real-time to protect both customers and the business.
Now that we have a solid understanding of predictive analytics letβs explore how itβs transforming the retail landscape.
Predictive Analytics in Retail: A Shopperβs Paradise 
The retail industry has quickly recognized the potential of predictive analytics and is leveraging it to create a shopping experience tailored to individual customers. Hereβs how itβs transforming the retail sector:
1. Personalized Product Recommendations
One of the most visible applications of predictive analytics in retail is personalized product recommendations. Youβve probably experienced this when shopping online, where a platform suggests similar products youβve viewed or purchased. This is made possible by predictive models that analyze your browsing and purchasing history to predict your interest.
These recommendations enhance the shopping experience by saving customers time and driving higher sales and customer loyalty. Retail giants like Amazon have perfected this art, using predictive analytics to recommend products and predict when youβre likely to run out of everyday items through subscription services like Amazon Subscribe & Save.
2. Inventory Optimization
Inventory management is a critical challenge for retailers. Stockouts can lead to missed sales opportunities, while excess inventory ties up capital and can result in financial losses. Predictive analytics helps retailers optimize their inventory by forecasting demand and automating restocking processes.
By analyzing historical sales data, seasonality, and external factors like weather, predictive models can determine when specific items will likely sell out. Retailers can then automatically reorder items, ensuring they are in stock when customers need them. Itβs a win-win situation, as customers get what they want when they want it, and retailers avoid stockouts.
3. Dynamic Pricing Strategies
Retailers are also using predictive analytics to implement dynamic pricing strategies. Instead of setting fixed prices for products, they adjust prices based on real-time market conditions, demand, and competitorsβ prices.
For example, airlines use dynamic pricing to adjust ticket prices based on factors such as the time of booking, seat availability, and even the customerβs browsing history. Similarly, online retailers can change prices based on the shopperβs behavior, location, and perceived willingness to pay.
Dynamic pricing allows retailers to maximize profits while remaining competitive in a fast-paced market. Itβs all made possible by
predictive analytics that continuously analyzes data and makes pricing recommendations in real time.
4. Customer Retention and Loyalty
Predictive analytics is a powerful tool for retaining customers and building brand loyalty. By analyzing customer behavior, retailers can identify signs of churn, such as reduced frequency of purchases or declining engagement.
Once potential churn is detected, retailers can take proactive measures, such as sending personalized offers, discounts, or loyalty program incentives to re-engage the customer. This prevents customers from leaving and fosters a sense of loyalty and appreciation.
5. Fraud Detection and Prevention
In the digital age, fraud has become a significant concern for online retailers. Predictive analytics is instrumental in identifying and preventing fraudulent transactions. By analyzing transaction data in real time and comparing it to historical patterns, predictive models can detect unusual behavior, flagging potentially fraudulent activities.
For example, if a customerβs credit card is suddenly used for a high-value transaction from a different location, the system can trigger an alert and request additional authentication. This protects the retailer from financial losses and ensures a secure shopping experience for customers.
Challenges and Considerations
While predictive analytics offers significant benefits to the retail industry, it also comes with its own set of challenges and considerations:
Data Quality
The accuracy of predictive models heavily relies on the quality of the data they are trained on. Retailers must ensure that the data used for predictions is clean, accurate, and up-to-date. This includes addressing missing data, duplicate entries, and inconsistencies.
Privacy and Ethics
Using customer data for predictive analytics raises essential privacy and ethical considerations. Retailers must be transparent about their data usage, obtain informed consent, and comply with data protection regulations like GDPR.
Model Interpretability
Complex machine learning models can sometimes be challenging to interpret. Retailers must strike a balance between model accuracy and the ability to explain how predictions are made, especially when dealing with sensitive decisions like pricing and credit approval.
Overfitting
Overfitting is a common challenge in predictive analytics, where a model is excessively tuned to the training data and performs poorly on new, unseen data. Retailers should use techniques like cross-validation to prevent overfitting and ensure model generalizability.
Data Security
Retailers must implement robust security measures to protect customer data from breaches and cyberattacks. Secure storage, encryption, and access controls are critical components of data security.
The Future of Retail: Data-Driven Shopping Experiences
As predictive analytics continues to evolve, the future of retail promises even more data-driven shopping experiences. Hereβs what we can expect:
Hyper-Personalization
Retailers will become even better at understanding individual customer preferences and needs. AI-powered chatbots and virtual shopping assistants will provide highly personalized guidance and product recommendations.
Augmented Reality (AR) Shopping
AR will take center stage in the retail world. Shoppers can virtually try on clothes, visualize furniture in their homes, and experience products before purchasing.
Sustainable Shopping
Predictive analytics will play a role in promoting sustainability. Retailers will use data to guide consumers toward eco-friendly choices, and predictive models will optimize supply chains to reduce waste.
Predictive Maintenance
Retailers with physical stores will implement predictive maintenance for equipment and facilities. Analyzing data from sensors and IoT devices allows them to schedule maintenance before issues arise, ensuring a seamless shopping experience.
Enhanced In-Store Analytics
Physical retail stores will leverage predictive analytics to gain insights into customer behavior. Heatmaps, dwell time analysis, and customer flow prediction will help retailers optimize store layouts and improve the customer experience.
Conclusion
Predictive analytics has unleashed a retail revolution, transforming the shopping experience for consumers and the operational efficiency of retailers. Retailers can anticipate customer needs, optimize inventory, set competitive prices, and build brand loyalty using historical data and sophisticated algorithms.
The future of retail is set to be data-driven, with even more personalized and immersive shopping experiences. As predictive analytics advances, online and physical retailers will have the tools to make data-informed decisions and stay competitive in the ever-evolving retail landscape.
So, whether youβre an avid shopper looking for the perfect product recommendations or a retailer seeking to enhance your customersβ experience, predictive analytics guides you through the aisles of the retail world, helping you make smarter, more informed choices.
Related Queries
Transforming Retail with Predictive Analytics
Retail Revolution
Boost Sales
Predictive Analytics Unveiled
Retail Success
Data-Driven Insights
Predictive Insights
AI and Retail
Retail Landscape
Sales Soaring
Save/Share this story with QR CODE
Disclaimer
This article is for informational purposes only and does not constitute endorsement of any specific technologies or methodologies and financial advice or endorsement of any specific products or services.
Need to get in touch?
Feel free to Contact NextGenDay.com for comments, suggestions, reviews, or anything else.
We appreciate your reading. Simple Ways To Say Thanks & Support Us:
1.) GIVE A TIP. Send a small donation thru Paypal
Your DONATION will be used to fund and maintain NEXTGENDAY.com
Subscribers in the Philippines can make donations to mobile number 0917 906 3081, thru GCash.
3.) BUY or SIGN UP to our AFFILIATE PARTNERS.
4.) Give this news article a THUMBS UP, and Leave a Comment (at Least Five Words).
AFFILIATE PARTNERS
World Class Nutritional Supplements - Buy Highest Quality Products, Purest Most Healthy Ingredients, Direct to your Door! Up to 90% OFF.
Join LiveGood Today - A company created to satisfy the world's most demanding leaders and entrepreneurs, with the best compensation plan today.