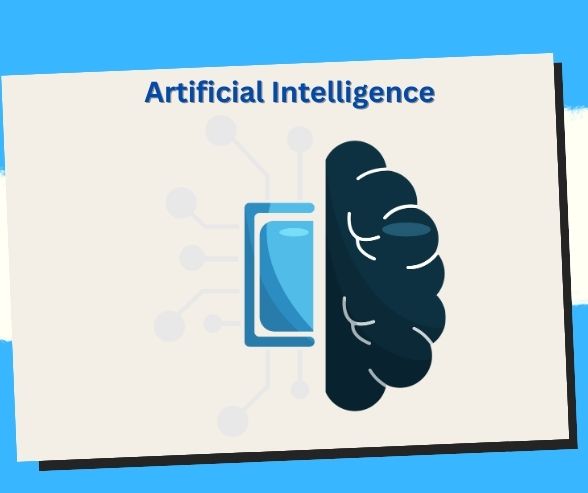
๐ฉโ๐ป Mastering AI Debiasing: Strategies for Fair and Ethical Development ๐คโ๏ธ
Explore strategies for mastering AI debiasing to ensure fairness and ethics in AI development. ๐คโ๏ธ #AIDebiasing #FairAI
Navigating Fair AI: A Deep Dive into Debiasing Techniques in AI Development ๐ค๐งน
Artificial Intelligence (AI) transforms how we live and work, from personalized recommendations to autonomous vehicles. However, the advent of AI has brought to light a critical concern: bias in AI algorithms. The unintentional introduction of bias can lead to unfair or discriminatory outcomes. In this article, we’ll explore the world of debiasing techniques in AI development, their implementation’s significance, and their role in creating more equitable AI systems. ๐๐ค
The Challenge of Bias in AI ๐คฏ
What is Bias in AI?
Bias in AI refers to systematic and unfair discrimination in the results and decisions made by AI systems. This bias can arise from the data used to train the models, algorithms, or user interactions. Bias can lead to unequal opportunities and reinforce existing societal inequalities.
The Impact of Bias on AI
The consequences of bias in AI can be profound:
- Criminal Justice: Biased algorithms can lead to unfair sentencing or risk assessment, perpetuating social disparities.
- Healthcare: Inaccurate diagnosis or treatment recommendations due to bias can result in unequal access to quality healthcare.
- Finance: Bias in lending or investment algorithms can lead to unequal access to financial resources.
The Quest for Debiasing in AI ๐
Debiasing in AI is mitigating bias to make AI systems fairer and more equitable. This involves recognizing and rectifying any bias in the data, algorithms, or decision-making processes. It’s a complex but crucial journey in AI development.
Identifying Bias ๐ง
Before diving into debiasing techniques, it’s essential to identify bias in AI systems. Here’s how to do it:
1. Data Analysis
- Data Auditing: Examine the training data for any underrepresented or overrepresented groups. Discrepancies can indicate potential bias.
- Data Distribution: Analyze data distribution across different categories, particularly sensitive attributes like gender, race, and age.
2. Outcome Disparities
- Comparison Across Groups: Evaluate how the algorithm’s outcomes differ across various groups. For example, in a hiring AI, check if it disproportionately rejects applications from specific demographics.
- Performance Metrics: Assess various performance metrics like accuracy, precision, and recall across different groups to identify discrepancies.
3. User Feedback
- User Complaints: User feedback can be invaluable in identifying bias, especially in recommendation systems or social media algorithms.
- Impact Assessment: Evaluate the real-world impact of the algorithm’s decisions to see if it disproportionately harms specific groups.
Strategies for Debiasing in AI ๐ ๏ธ
The path to debiasing AI involves multiple strategies. Let’s explore the techniques and methods used to mitigate bias:
1. Preprocessing
Data Cleaning: Remove biased or incorrect data entries to create a more balanced dataset.
Data Augmentation: Introduce new data points or transform existing ones to increase diversity in the training data.
2. Fair Algorithm Design
Algorithmic Adjustments: Modify the algorithm to account for bias. Techniques like re-weighting or re-sampling can give more importance to underrepresented groups.
Feature Engineering: Carefully select, or engineer features to reduce the impact of sensitive attributes like gender or race.
3. Regular Auditing
Continuous Monitoring: Implement continuous monitoring to detect and address emerging bias. Re-evaluate data, outcomes, and user feedback periodically.
User-Centric Approach: Involve users actively in auditing to gather insights and refine algorithms.
4. Ethical Considerations
Ethics Review: Perform ethical reviews of algorithms before deployment to assess potential impacts on vulnerable or underrepresented groups.
Transparency: Be transparent about the decision-making process of your algorithms, and share information on how they work and steps taken to mitigate bias.
5. Diverse Development Teams
Inclusive Teams: Build diverse development teams that bring varied perspectives and experiences. Diverse voices can help uncover and rectify potential bias during the design phase.
External Auditors: Consider external audits of your algorithms to gain an unbiased perspective on their fairness.
Domain-Specific Debiasing ๐
Bias in AI is not uniform across all domains. It manifests differently and requires tailored debiasing techniques. Let’s explore some key sectors:
1. Healthcare
In healthcare, AI aids in diagnosis and treatment recommendations. Debiasing in healthcare AI ensures that models are equally proficient at providing accurate advice to diverse patient populations.
2. Criminal Justice
AI systems in criminal justice assist with risk assessment, bail decisions, and sentencing. Debiasing is essential to prevent algorithms from exacerbating existing inequalities in the justice system.
3. Finance
AI models determine lending decisions, credit scores, and investment recommendations in finance. Debiasing is crucial to prevent discrimination in lending and access to financial resources.
4. Education
AI is making its mark in education through personalized learning platforms. Debiasing in education AI ensures that these systems provide equitable recommendations to students from diverse backgrounds.
The Role of Regulations and Standards ๐
Regulatory bodies and industry standards play a crucial role in promoting debiasing in AI:
GDPR and Data Protection
The General Data Protection Regulation (GDPR) in Europe strongly emphasizes data protection and individual rights. GDPR encourages organizations to ensure fair representation and debiasing in AI while respecting privacy.
AI Ethics Guidelines
Organizations like the IEEE and the Partnership on AI have developed AI ethics guidelines emphasizing debiasing’s importance. These guidelines stress the need for transparency and equity in AI systems.
Federal Legislation
Several countries, including the United States, are exploring or enacting AI and data fairness legislation. These regulations aim to establish standards for the responsible use of AI, ensuring equitable representation.
The Road Ahead: Ethical AI for All ๐
As technology continues to shape our world, we must strive for fairness and equity in AI systems. The journey toward creating a more ethical AI landscape is ongoing and dynamic.
Technological Advances
Technological advancements in AI are aiding the identification and mitigation of bias. Machine learning models are improving at recognizing and addressing bias in training data.
Public Awareness
Growing public awareness of the importance of fair and ethical AI drives discussions and demands transparency and fairness. This collective awareness is a powerful force for holding organizations and developers accountable.
Ethical AI
The field of ethical AI is rapidly evolving, with researchers and ethicists actively working on innovative techniques and frameworks to address bias. Ethical considerations are becoming an integral part of AI development.
Collaborative Efforts
Stakeholders from all sectors, including governments, businesses, researchers, and advocacy groups, recognize collaboration’s importance in addressing bias in AI. Joint efforts are essential to creating a more equitable AI landscape.
In Conclusion: A Brighter, Fairer AI Future ๐
Debiasing in AI is a complex but vital mission. As technology continues to influence our lives, we must navigate the path with a commitment to transparency, accountability, and fairness.
The road to a more ethical AI future is long and winding but a journey worth taking. Let us strive for an AI landscape that empowers and uplifts all individuals, regardless of their background or characteristics, fostering a more just and equitable world. ๐๐ค๐
Related Queries
Mastering Debiasing in AI Development
Top Techniques for Ethical AI Development
Navigating Uncharted Territory in Debiasing AI
From Bias to Balance: Debiasing AI Algorithms
Shaping the Future with Ethical AI Debiasing
Achieving Algorithmic Equity through Precision Debiasing
Empowering Ethical AI with Debiasing
Guidance on Successful Debiasing in AI
Cornerstones of AI Fairness: Data and Model Debiasing
Purposeful Debiasing for Ethical Algorithms
Save/Share this story with QR CODE
Disclaimer
This article is for informational purposes only and does not constitute endorsement of any specific technologies or methodologies and financial advice or endorsement of any specific products or services.
๐ฉ Need to get in touch?
Feel free to Email Us for comments, suggestions, reviews, or anything else.
We appreciate your reading. ๐Simple Ways To Say Thanks & Support Us:
1.) โค๏ธGIVE A TIP. Send a small donation thru Paypal๐โค๏ธ
Your DONATION will be used to fund and maintain NEXTGENDAY.com
Subscribers in the Philippines can make donations to mobile number 0917 906 3081, thru GCash.
3.) ๐ BUY or SIGN UP to our AFFILIATE PARTNERS.
4.) ๐ Give this news article a THUMBS UP, and Leave a Comment (at Least Five Words).
AFFILIATE PARTNERS
World Class Nutritional Supplements - Buy Highest Quality Products, Purest Most Healthy Ingredients, Direct to your Door! Up to 90% OFF.
Join LiveGood Today - A company created to satisfy the world's most demanding leaders and entrepreneurs, with the best compensation plan today.