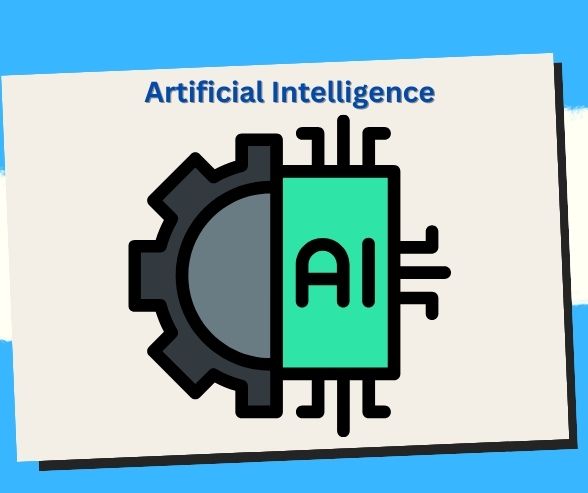
โ๏ธ Detecting Algorithmic Bias: Tools and Strategies for Transparency ๐
Explore how artificial intelligence is revolutionizing our understanding of algorithmic bias and driving change.
Unveiling the Veil of Algorithmic Bias with Artificial Intelligence ๐ค
In our ever-evolving digital landscape, algorithms play an increasingly significant role in decision-making processes. From social media feeds to loan approvals, algorithms wield substantial power in shaping our experiences and opportunities. However, there’s a growing concern regarding algorithmic bias โ the potential for these algorithms to discriminate against certain groups. But fear not! With the help of artificial intelligence (AI), we can shed light on this issue and work towards creating fairer systems for all.
Understanding Algorithmic Bias
Before delving into how AI can combat algorithmic bias, it’s crucial to understand what exactly algorithmic bias is. Essentially, algorithmic bias occurs when an algorithm produces results that systematically disadvantage or advantage certain groups of people. This bias can stem from various sources, including historical data, programmer bias, or inherent limitations in the algorithm’s design.
Identifying Bias with AI
One of the most powerful tools in our arsenal for combating algorithmic bias is AI itself. Machine learning algorithms can be trained to recognize patterns of bias within datasets and algorithms. By analyzing vast amounts of data, AI algorithms can pinpoint disparities in outcomes and highlight areas where bias may be present.
๐ Tip 1: Utilize AI-powered auditing tools to assess algorithms for potential biases. These tools can provide valuable insights into the inner workings of algorithms and help identify areas for improvement.
๐ Tip 2: Implement diverse datasets during the training phase of algorithm development. By incorporating data from a wide range of sources and demographics, we can reduce the risk of bias in the resulting algorithms.
Mitigating Bias in Algorithm Design
Once bias has been identified, it’s essential to take proactive steps to mitigate its effects. This involves examining every stage of the algorithm’s lifecycle, from data collection to model deployment.
๐ ๏ธ Tip 3: Employ fairness-aware algorithms that are specifically designed to minimize bias and promote equitable outcomes. These algorithms incorporate fairness constraints into their optimization process, ensuring that all individuals are treated fairly.
๐ Tip 4: Regularly monitor and update algorithms to adapt to changing societal norms and contexts. What may be considered fair today may not be the case tomorrow, so it’s crucial to stay vigilant and responsive to evolving standards.
Promoting Transparency and Accountability
Transparency is key to building trust in algorithmic systems. By making the inner workings of algorithms accessible to the public, we can hold developers and organizations accountable for any biases present in their systems.
๐ Tip 5: Implement transparency measures such as algorithmic impact assessments and documentation of decision-making processes. This not only fosters accountability but also enables stakeholders to understand how algorithms may affect them.
๐ข Tip 6: Foster open dialogue and collaboration between stakeholders, including data scientists, policymakers, and affected communities. By engaging in meaningful conversations, we can collectively work towards creating more inclusive and equitable algorithmic systems.
The Importance of Addressing Algorithmic Bias
Addressing algorithmic bias is crucial for several reasons:
1. Promoting Fairness
Biased algorithms can lead to discriminatory outcomes, affecting individuals’ access to opportunities and resources unfairly.
2. Enhancing Trust
Reducing bias in AI systems fosters trust among users and stakeholders, encouraging broader adoption and acceptance of AI technologies.
3. Ensuring Accountability
By identifying and mitigating bias, organizations can uphold ethical standards and mitigate legal risks associated with discriminatory practices.
4. Improving Decision-Making
Unbiased algorithms enable more accurate and reliable decision-making, enhancing the quality of outcomes across various domains.
5. Advancing Social Justice
Addressing algorithmic bias contributes to the pursuit of social justice by reducing disparities and promoting equality of opportunity.
6. Fostering Innovation
Fair and inclusive AI systems foster innovation by leveraging diverse perspectives and minimizing the potential for discriminatory practices.
7. Enhancing Diversity
Mitigating bias in AI promotes diversity and inclusion by ensuring that algorithms account for the needs and experiences of all individuals.
8. Supporting Economic Growth
Fair AI systems can drive economic growth by facilitating equal access to opportunities and resources for all segments of society.
9. Protecting Human Rights
By reducing discriminatory outcomes, efforts to address algorithmic bias uphold fundamental human rights and dignity.
10. Building a Better Future
Ultimately, tackling algorithmic bias contributes to building a more equitable and just society for future generations.
Case Studies: Uncovering Bias in AI Systems
1. Bias in Facial Recognition
Research has revealed significant biases in facial recognition systems, with higher error rates for certain demographic groups, particularly people of color and women.
2. Biased Hiring Algorithms
Several companies have faced scrutiny for biased hiring algorithms that perpetuate gender or racial disparities, leading to discrimination against qualified candidates.
3. Discriminatory Loan Approvals
AI-driven lending algorithms have been found to disproportionately deny loans to minority applicants, perpetuating economic inequality and hindering financial inclusion.
4. Healthcare Disparities
Healthcare algorithms have exhibited biases in diagnosis and treatment recommendations, leading to disparities in access to care and health outcomes among different demographic groups.
5. Criminal Justice Biases
Predictive policing algorithms have been criticized for perpetuating racial profiling and exacerbating disparities in law enforcement practices and criminal justice outcomes.
6. Biases in Online Advertising
Algorithmic advertising platforms have been accused of perpetuating stereotypes and discrimination by targeting or excluding certain demographic groups based on sensitive attributes.
7. Educational Inequities
AI-powered educational tools may inadvertently reinforce existing educational inequities by failing to account for diverse learning needs and backgrounds.
8. Biases in Financial Services
Algorithmic trading systems and credit scoring models have been found to exhibit biases that disadvantage certain demographic groups in access to financial services and opportunities.
9. Autonomous Vehicles and Safety
Concerns have been raised about the potential biases in algorithms governing autonomous vehicles, particularly regarding pedestrian detection and risk assessment.
10. Social Media Echo Chambers
Algorithmic content recommendation systems on social media platforms can reinforce echo chambers and filter bubbles, amplifying biases and polarizing discourse.
Key Takeaways
- Awareness is Key: Recognizing the existence and implications of algorithmic bias is the first step towards addressing it effectively.
- Diverse Data Matters: Ensuring diversity and representativeness in training data is essential for building unbiased AI models.
- Transparency Builds Trust: Transparent algorithms and decision-making processes enhance accountability and trustworthiness.
- Continuous Evaluation is Necessary: Regular monitoring and evaluation of AI systems are crucial for identifying and mitigating bias over time.
- Ethical Guidelines are Essential: Developing and adhering to ethical guidelines can help guide the responsible development and deployment of AI technologies.
- Interdisciplinary Collaboration is Valuable: Collaboration between data scientists, ethicists, social scientists, and other stakeholders can enrich discussions and solutions for addressing algorithmic bias.
- Bias Mitigation Requires Contextual Understanding: Contextual understanding of the social, cultural, and historical factors at play is necessary for effective bias mitigation strategies.
- User Feedback is Critical: Soliciting feedback from diverse user groups can help identify biases and improve the fairness and inclusivity of AI systems.
- Regulatory Oversight is Important: Governments and regulatory bodies play a vital role in setting standards and regulations to ensure fairness and accountability in AI deployment.
- Bias Mitigation is a Continuous Process: Eliminating algorithmic bias is an ongoing journey that requires commitment, vigilance, and collaboration across disciplines and industries.
Frequently Asked Questions (FAQs)
1. What causes algorithmic bias?
Algorithmic bias can result from various factors, including biased training data, flawed algorithms, and the lack of diversity in development teams.
2. How can organizations detect algorithmic bias in their AI systems?
Organizations can use various techniques, such as fairness metrics, bias audits, and user testing, to detect and mitigate algorithmic bias in their AI systems.
3. Can AI algorithms be completely unbiased?
Achieving complete neutrality in AI algorithms may be challenging due to the inherent complexity of human society and decision-making. However, efforts can be made to reduce and mitigate bias to the greatest extent possible.
4. How can bias be mitigated in AI systems?
Bias mitigation strategies may include diverse data collection, algorithmic transparency, fairness-aware algorithms, bias audits, and user feedback mechanisms.
5. What role do policymakers play in addressing algorithmic bias?
Policymakers can enact regulations and guidelines to promote fairness, transparency, and accountability in AI deployment, thereby addressing algorithmic bias and ensuring ethical AI practices.
6. Are there ethical considerations associated with using AI to mitigate bias?
Yes, ethical considerations such as privacy protection, consent, and fairness must be carefully considered when implementing AI-based bias mitigation strategies.
7. How can AI developers promote diversity and inclusion in their teams?
AI developers can promote diversity and inclusion by actively recruiting from underrepresented groups, fostering inclusive work environments, and incorporating diverse perspectives into the development process.
8. Are there any legal implications associated with algorithmic bias?
Yes, organizations that deploy biased AI systems may face legal consequences, including lawsuits, regulatory fines, and damage to reputation and trust.
9. Can AI algorithms perpetuate existing societal inequalities?
Yes, if not properly designed and implemented, AI algorithms can perpetuate existing societal inequalities by replicating and amplifying biases present in historical data and societal structures.
10. How can individuals advocate for fair and unbiased AI?
Individuals can advocate for fair and unbiased AI by raising awareness about algorithmic bias, supporting initiatives for diversity and inclusion in AI development, and holding organizations and policymakers accountable for ethical AI practices.
Conclusion
Unveiling the veil of algorithmic bias with artificial intelligence is a multifaceted challenge that requires collective effort and interdisciplinary collaboration. By understanding the causes and consequences of algorithmic bias, implementing mitigation strategies, and upholding ethical principles, we can harness the power of AI to foster fairness, inclusivity, and social justice in the digital age. As we navigate this complex terrain, it is imperative to remain vigilant, proactive, and committed to building AI systems that reflect our values and aspirations for a better future.
Remember, the journey towards unbiased AI is ongoing, but every step we take brings us closer to realizing the transformative potential of artificial intelligence for the benefit of all.
Key Phrases
- Algorithmic bias detection
- Artificial intelligence fairness
- Uncovering hidden discrimination
- Systemic bias revelation
- Fighting algorithmic injustice
- AI-driven equity initiatives
- Discrimination mitigation with AI
- Fair decision-making algorithms
- Bias-aware AI technologies
- Promoting algorithmic transparency
Hashtags
- #AlgorithmicBias
- #AIForEquality
- #FairAI
- #UnveilingDiscrimination
- #EthicalAlgorithms
- #BiasDetection
- #TransparentAI
- #AlgorithmicFairness
- #InclusiveTech
- #AIJustice
Save/Share this story with QR CODE
Disclaimer
This article is for informational purposes only and does not constitute endorsement of any specific technologies or methodologies and financial advice or endorsement of any specific products or services.
๐ฉ Need to get in touch?
๐ฉ Feel free to Contact NextGenDay.com for comments, suggestions, reviews, or anything else.
We appreciate your reading. ๐Simple Ways To Say Thanks & Support Us:
1.) โค๏ธGIVE A TIP. Send a small donation thru Paypal๐โค๏ธ
Your DONATION will be used to fund and maintain NEXTGENDAY.com
Subscribers in the Philippines can make donations to mobile number 0917 906 3081, thru GCash.
3.) ๐ BUY or SIGN UP to our AFFILIATE PARTNERS.
4.) ๐ Give this news article a THUMBS UP, and Leave a Comment (at Least Five Words).
AFFILIATE PARTNERS
World Class Nutritional Supplements - Buy Highest Quality Products, Purest Most Healthy Ingredients, Direct to your Door! Up to 90% OFF.
Join LiveGood Today - A company created to satisfy the world's most demanding leaders and entrepreneurs, with the best compensation plan today.