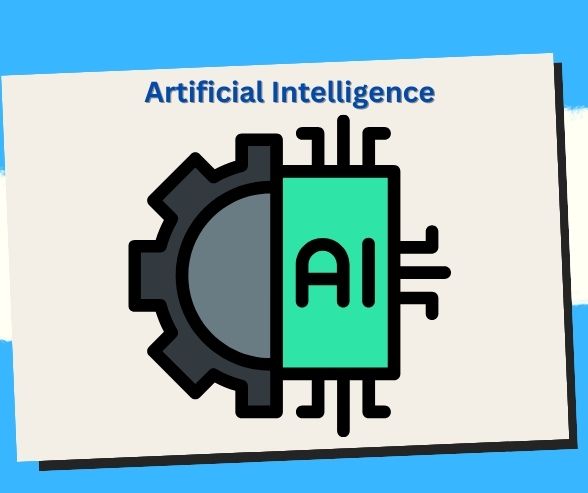
Cracking the Code: Strategies for Addressing the Black Box Problem in AI
Step out of the shadows with our guide! Discover solutions to the black box dilemma in AI, unveiling strategies that bring transparency and clarity to the complex algorithms driving artificial intelligence.
Cracking Open the Black Box: Addressing the Mysteries of AI with Transparency 🌐🕵️
Unveiling the Shadows – The Black Box Problem in AI
In the realm of Artificial Intelligence (AI), where algorithms make decisions that impact our lives, the “black box problem” looms large. This blog post embarks on a journey to shine a light into the shadows, exploring the intricacies of the black box problem in AI, its significance, challenges, and the imperative of addressing the mysteries within.
The Veiled Enigma: Understanding the Black Box Problem in AI 🎭🕵️
The Opacity of Algorithmic Decision-Making
The black box problem in AI refers to the opacity surrounding how algorithms arrive at specific decisions. In many cases, even the creators of these algorithms struggle to decipher the intricate processes within the algorithmic black box. This lack of transparency raises concerns about accountability, biases, and ethical implications.
Why the Black Box Matters
Understanding the black box is not just a technical necessity; it’s a matter of trust and accountability. As AI becomes an integral part of our daily lives, from recommendation systems to autonomous vehicles, knowing how and why AI systems make decisions is crucial for users, regulators, and developers alike.
Peeling Back the Layers: Significance of Addressing the Black Box Problem 🌐🔍
Building Trust Through Transparency
Addressing the black box problem is synonymous with building trust. Transparency allows users to comprehend the decision-making processes of AI systems, fostering a sense of trust and confidence in the technology.
Ensuring Accountability in AI Systems
Transparency is a cornerstone of accountability. If something goes awry in an AI system, whether it’s biased decisions or unforeseen errors, understanding the inner workings enables accountability. It’s about knowing who or what is responsible for the decisions made.
The Challenges of Opacity: Navigating the Complexity of the Black Box 🌊🧩
Balancing Confidentiality and Transparency
One of the inherent challenges is finding the balance between maintaining the confidentiality of proprietary algorithms and providing enough transparency for accountability. Striking this delicate balance requires careful consideration of ethical, legal, and business aspects.
The Dangers of Hidden Biases
Hidden biases within AI systems are amplified by the black box problem. Biases in training data or algorithmic decision-making can perpetuate discrimination. Addressing the black box problem is, therefore, a step towards identifying and rectifying biased outcomes.
Strategies for Illumination: Tackling the Black Box Problem Head-On 🚀🔦
Explainable AI Models
Adopting explainable AI models is a strategic move to tackle the black box problem. These models are designed to provide clear insights into the decision-making processes of algorithms, making them more interpretable for users and stakeholders.
Auditing and Open-Source Initiatives
Regular auditing of AI systems and embracing open-source initiatives contribute to addressing the black box problem. Audits provide an external perspective on system behavior, while open-source initiatives encourage collaborative scrutiny and understanding.
The Ethical Imperative: Addressing the Black Box as a Moral Duty 🌐🤔
Ensuring Fair and Transparent AI Systems
Addressing the black box problem is not just a technical challenge; it’s a moral duty. Transparent AI systems are essential for ensuring fairness, preventing discrimination, and upholding ethical standards in the deployment of AI technologies.
User Empowerment Through Understanding
Demystifying the black box empowers users. When users understand how AI systems function, they can make informed decisions, question outcomes, and actively engage with technology. This empowerment is a key outcome of addressing the black box problem.
Top 10 Best Resources about the Black Box Problem in AI
1. Explainable AI (XAI) Initiative
https://www.darpa.mil/program/explainable-artificial-intelligence
DARPA’s XAI program focuses on developing methods for making AI models more understandable and interpretable. This website provides information on research projects, challenges, and events related to XAI.
2. Blackbox AI: A Review and Survey of Explainable Artificial Intelligence Techniques
https://arxiv.org/pdf/2210.05173
This academic paper provides a comprehensive overview of the black box problem and various XAI techniques for addressing it.
3. FAT (Fairness, Accountability, Transparency) in Sociotechnical Systems:
https://dl.acm.org/doi/proceedings/10.1145/3287560
This annual conference brings together researchers and practitioners to discuss issues related to fairness, accountability, and transparency in AI and other sociotechnical systems.
4. Towards Demystifying AI for Social Good
https://www.nature.com/articles/s41467-020-15871-z
This website features resources and research projects on various aspects of responsible AI, including demystifying black box models for social good applications.
5. The Algorithmic Justice League (AJL)
AJL advocates for a just and equitable world by challenging bias and discrimination in algorithms. Their website includes resources on the black box problem and its implications for fairness and accountability.
6. ProGAN: Generating and Understanding Deep Images
This research paper introduces ProGAN, a generative adversarial network (GAN) with improved interpretability features, allowing for a better understanding of how the model generates images.
7. SHAP (SHapley Additive exPlanations)
SHAP is a popular package for explaining individual predictions made by machine learning models, providing insights into their decision-making process.
8. Interpretable Machine Learning with LIME
https://christophm.github.io/interpretable-ml-book/lime.html
LIME (Local Interpretable Model-Agnostic Explanations) is another technique for explaining individual predictions, offering model-agnostic interpretations applicable to various models.
9. MIT Technology Review: The Black Box Problem in AI
https://news.mit.edu/2023/stefanie-jegelka-machine-learning-0108
This article from MIT Technology Review explores the challenges of the black box problem and its implications for various domains.
10. Explainable AI (XAI) News
https://www.reuters.com/technology/elon-musks-xai-files-raise-up-1-bln-equity-offering-2023-12-05/
This website aggregates news and research articles related to Explainable AI and the black box problem, offering a continually updated resource for staying informed.
These resources provide valuable insights into the black box problem in AI and the ongoing efforts to develop solutions for greater transparency and interpretability. Remember, addressing the black box problem is crucial for building trust and ensuring responsible development and deployment of AI systems.
Conclusion: Illuminating the AI Path with Transparency 🌟🔍
In the intricate dance between humans and algorithms, addressing the black box problem is not just a technical challenge but a moral imperative. As we journey into a future where AI plays an increasingly pervasive role, let us champion the cause of transparency, illuminating the AI path with understanding, trust, and ethical responsibility. The quest to address the black box problem is a journey towards a future where the mysteries of AI are unveiled, and technology serves as a beacon of fairness, accountability, and empowerment.
Key Phrases to Remember
- Black Box Problem in AI
- Transparency in Algorithmic Decision-Making
- Building Trust Through Transparency
- Ensuring Accountability in AI Systems
- Balancing Confidentiality and Transparency
- Dangers of Hidden Biases in AI
- Explainable AI Models for Interpretability
- Auditing AI Systems for Transparency
- Addressing the Black Box as a Moral Duty
- User Empowerment Through Understanding
Best Hashtags
- #AItransparency
- #BlackBoxProblem
- #EthicalAI
- #AlgorithmicAccountability
- #ExplainableAI
- #TechEthics
- #UserEmpowerment
- #OpenSourceAI
- #AuditingAlgorithms
- #FairAI
Save/Share this story with QR CODE
Disclaimer
This article is for informational purposes only and does not constitute endorsement of any specific technologies or methodologies and financial advice or endorsement of any specific products or services.
📩 Need to get in touch?
📩 Feel free to Contact NextGenDay.com for comments, suggestions, reviews, or anything else.
We appreciate your reading. 😊Simple Ways To Say Thanks & Support Us:
1.) ❤️GIVE A TIP. Send a small donation thru Paypal😊❤️
Your DONATION will be used to fund and maintain NEXTGENDAY.com
Subscribers in the Philippines can make donations to mobile number 0917 906 3081, thru GCash.
3.) 🛒 BUY or SIGN UP to our AFFILIATE PARTNERS.
4.) 👍 Give this news article a THUMBS UP, and Leave a Comment (at Least Five Words).
AFFILIATE PARTNERS
World Class Nutritional Supplements - Buy Highest Quality Products, Purest Most Healthy Ingredients, Direct to your Door! Up to 90% OFF.
Join LiveGood Today - A company created to satisfy the world's most demanding leaders and entrepreneurs, with the best compensation plan today.